Stora Enso develops production processes through machine learning with CollectiveCrunch
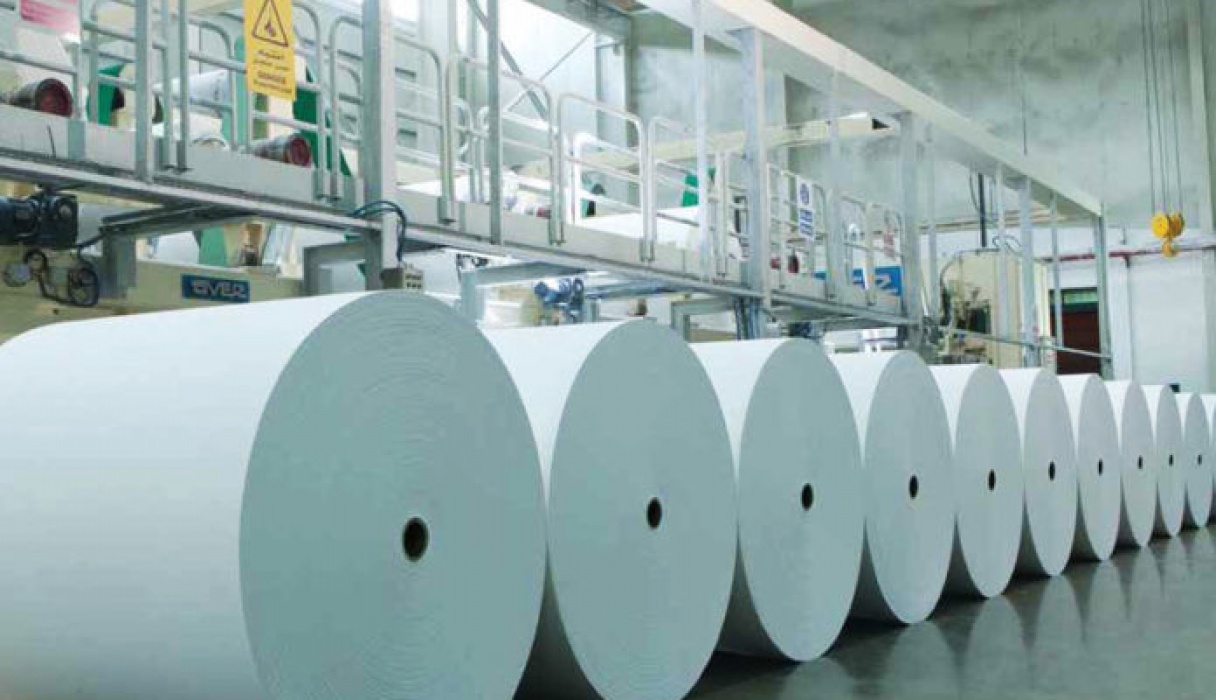
Takeoff Partners' portfolio company CollectiveCrunch takes part in Stora Enso's startup program. The paper company's ambition is to save millions of euros annually through improved prediction of the costly cracks to the paper belt during the production process.
"Paper making is about constantly balancing for instance the humidity levels", describes Stora Enso's Otto Mertanen in the article by Kauppalehti (originally in Finnish). "No-one really knows why the band breaks up since there are 150,000 parameters that can have an influence on it."
It's not an insignificant problem; Stora Enso's paper division alone takes a 40 million euro financial hit each year because the entire factory needs to be restarted after a paper band breaks up.
Traditionally, large enterprises have developed solutions in secret, yet this time Stora Enso decided to open up the solution seeking and involve startups in the process.
With CollectiveCrunch, Stora Enso developed an ERP add-on named "Linda" (Learning Intelligent Network Data Agent). Linda analyzes the parameters that may cause cracks in the paper belt and sends signals to the paper mill employees when the paper is about to crack. With the help of machine learning, Linda develops over time and finds the cause-and-effect relationships related to cracks increasingly well.
Efficiency in asset management through new technology
CollectiveCrunch enables a change of paradigm for industrial companies in understanding and managing their assets and systems that are exposed to the natural environment.
In practice, CollectiveCrunch is a data and analytics platform that uses machine learning algorithms to put together and interpret data from two sources:
1) Predictions (historical or current) on the natural environment, and
2) Company owned historical data (mainly data from previous years)
For companies whose business is exposed to fluctuations in the natural environment, the potential financial impact of more accurate forecasting is massive: When volumes are very large, even a 1% systematic improvement in forecast accuracy will result in saving the company several millions of euros annually.
The large-scale use of historical or current data from technical, climate and geo sources, together with algorithms and AI, is unforeseen. The predictions that companies use today are mainly based on in-house statistics. The utilization of machine learning will improve the forecasting process and its outcomes drastically.
ERP systems miss the prediction layer
“The current ERP systems do not offer this level of forecasting tools even though anticipating the use of machinery and equipment is an integral part of the resource planning in companies. The ‘prediction layer’ is missing”, says Jukka Sonninen, Chairman of the Board of CollectiveCrunch and the former head of SAP Finland.
“A major driver behind the need for better forecasting tools is the constantly evolving natural environment that makes it harder for many businesses to plan for the use of their assets on a daily basis”, Sonninen continues.